AI is transforming the way medicine approaches personalized healthcare, particularly through genomics. By analyzing vast genetic data, AI helps clinicians identify potential health risks, predict disease outcomes, and create individualized treatment plans.
The fusion of AI and genomics is invaluable in improving patient outcomes, making precision medicine more accessible and effective. With the growing demand for personalized healthcare solutions, AI in genomics is becoming a crucial element in modern medical practices.
The global AI in genomics market experienced a significant increase from $0.75 billion in 2023 to $1.08 billion in 2024, with a projected CAGR of 43.9% to $4.58 billion in 2028.
FHIR plays an important role in integrating genomic data into healthcare systems. Traditionally, genomics data has been challenging to incorporate into EHRs due to the complexity and volume of information. Current practices often rely on unstructured, static reports that do not effectively assist clinicians.
FHIR offers a structured approach to solve these limitations, allowing genomic information to be easily shared and analyzed. Although it presents challenges, including data variability and interoperability issues, FHIR Genomics is gaining traction and is a promising solution for integrating vast genomic datasets into clinical workflows.
Key Genomic Components in FHIR
Understanding the key genomic components in FHIR is essential for effective data exchange and integration in healthcare applications. Here are a few points to consider:
🧬 Genetic Variants
Genetic variants are changes in the DNA sequence that can influence an individual's health. These alterations come in various forms, such as single nucleotide polymorphisms (SNPs), insertions, or deletions. SNPs are small changes in a single base pair, while insertions or deletions can affect larger segments of DNA. These variants can impact how genes are expressed and how proteins function, which is key in understanding the risk of certain diseases. In the context of AI and genomics, analyzing these variants through FHIR data enables personalized treatment decisions in healthcare.
🧬 Molecular Sequences
Molecular sequences refer to how genetic variants influence biological processes, such as protein production or gene expression. For example, some variants may disrupt protein folding or interfere with splicing, impacting a cell's ability to function normally. These disruptions can lead to conditions like cancer, cardiovascular diseases, or neurological disorders. When integrated with FHIR data, AI can dig deep into molecular details to better understand health outcomes. It helps healthcare providers fine-tune treatments to address specific molecular issues in patients, allowing for a more personalized and effective approach to care.
AI-Driven Use Case Scenarios
Discover practical AI-driven use case scenarios across different industries; let's discuss it below.
1️⃣ Personalized Treatment Planning
Analyzing genetic variants helps design more individualized treatment plans. AI models can evaluate a patient's unique genetic makeup, identifying specific reactions to medications or therapies.
For example, data from genetic tests may show whether a patient metabolizes drugs faster or slower, leading to a more precise dosage recommendation. This targeted approach reduces trial-and-error treatments, improving patient outcomes and treatment efficiency. Incorporating such insights into healthcare allows providers to offer more accurate and tailored medical care, aligning treatment with the patient's genetic profile.
2️⃣ Risk Assessment and Early Intervention
Assessing genetic variants allows for earlier identification of potential health risks. Predictive models analyze a person’s genetic profile, revealing predispositions to conditions such as cardiovascular disease, diabetes, or cancer. By recognizing these risks before symptoms emerge, healthcare providers can recommend preventive measures such as lifestyle changes or early interventions.
This proactive approach to healthcare reduces the likelihood of serious disease development and improves patient engagement, encouraging long-term health management tailored to individual genetic predispositions.
3️⃣ Precision Drug Response Prediction
Predicting drug efficacy based on genetic information ensures that medications are more effective and safer for individual patients. Certain genetic variants can influence how a patient responds to specific drugs, affecting both efficacy and the likelihood of adverse reactions.
Using AI-driven analysis, healthcare professionals can predict whether a patient will benefit from a particular drug or require dosage adjustments. It reduces the risk of complications and minimizes unnecessary side effects, ensuring that each prescription aligns with the patient's genetic profile, leading to more successful and efficient treatments.
4️⃣ Molecular Diagnostics and Prognostics
The ability to interpret molecular sequences of genetic variants aids in diagnosing and predicting the course of diseases. By analyzing how certain genetic changes affect molecular processes like protein function, healthcare providers gain valuable insights into disease mechanisms. This information can inform decisions on therapeutic targets, allowing for more precise interventions.
In cases where genetic variants are linked to specific diseases, understanding their molecular sequences helps guide treatment strategies, offering a clearer prognosis and better patient outcomes. The integrated approach enhances the precision of diagnostics and therapeutic decision-making.
Related Read: Benefits of Interoperable Healthcare Data for Patients
Value Creation for Key Players
Understanding how AI drives value creation is essential for stakeholders. AI enhances efficiency, boosts productivity, and supports informed decision-making.
✅ For Patients
Patients benefit from more personalized treatment plans that align with their unique genetic profiles. By utilizing genetic data, healthcare providers can identify early risk factors, leading to proactive management of potential health issues. This approach reduces the need for trial-and-error in medication, as treatments are more likely to be effective. The inclusion of genomics data enables patients to avoid adverse reactions and experience better health outcomes. The use of AI in genomics has opened doors for more precise care, allowing individuals to receive treatments tailored to their genetic makeup, significantly improving medication efficacy.
✅ For Doctors
Doctors gain valuable insights into patient care through the integration of FHIR and genomics. With access to genetic data, physicians can make informed decisions more quickly. Complex analyses of genetic variants and their molecular sequences become more manageable, reducing the time spent on manual interpretations. Faster diagnosis allows doctors to focus more on patient care and treatment plans. AI in genomics supports better decision-making and streamlines workflows, leading to improved efficiency in the medical field. The ability to predict disease risks or responses to medications makes clinical decisions more precise.
✅ For Payers/Insurance Companies
Payers and insurance companies experience significant cost savings by focusing on data-driven healthcare. Treatments guided by genetic insights are more effective, reducing the overall cost of unnecessary or ineffective procedures. Fewer claim disputes arise as the decision-making process becomes clearer, with treatments backed by genomic data showing their potential efficacy. AI in genomics supports population health management by identifying high-risk individuals early, allowing for preventative measures that lower long-term healthcare costs. This approach ensures that insurance companies can provide coverage more efficiently while maintaining better patient outcomes.
Related read: FHIR vs HL7: The Battle for Interoperability in Cloud-Based Healthcare
Challenges in Integrating AI with Genomics
Integrating AI with genomics presents unique challenges that can impact the effectiveness of applications in healthcare and research. Here are key obstacles:
❗Data Privacy
Protecting genetic data remains a significant challenge. Genetic information is highly sensitive, and any breach could expose personal health details. The risk of misuse or unauthorized access heightens privacy concerns. As AI tools become more common in genomics, they require stringent security protocols to safeguard this valuable data. Maintaining compliance with regulatory requirements, such as HIPAA, while managing vast volumes of genomic data adds complexity. Addressing privacy risks remains essential as more healthcare providers seek to integrate AI-powered genomics solutions into their workflows.
❗Ethical Considerations
Using AI in genomics presents ethical dilemmas. Genetic data can reveal information about the patient and their relatives, raising concerns about consent and privacy. Ethical issues like discrimination, particularly in insurance or employment, based on genetic predispositions, arise. Further, the use of AI to interpret genetic data can lead to unintended biases, as AI models are trained on data that may not represent all populations equally. Establishing clear guidelines to ethically manage genetic data usage is crucial in mitigating these concerns as the genomics market continues to grow.
❗Integration Complexity
Integrating AI into existing healthcare systems introduces technical hurdles. AI models need to seamlessly integrate with clinical workflows without disrupting care. Genomics-EHR integration, for example, faces challenges due to the volume and complexity of genomic data. Moreover, EHR systems must be upgraded to handle structured genomic data, requiring collaboration between EHR vendors and AI developers. Overcoming integration challenges is key to making AI in genomics more practical for clinical use.
❗Genomics-EHR Integration Challenges
Current genomic reports delivered through EHRs often contain fragmented data that lacks structure, making it difficult for clinicians to use. EHR systems typically integrate genomic test results, which provide limited insights and are not ideal for real-time clinical decision-making. Structured genomic data is needed to improve clinical decision support (CDS) tools and analytics. Despite advancements like HL7 FHIR standards, effective integration remains elusive. The sheer volume and complexity of next-generation sequencing (NGS) data, alongside limited interoperability between data formats, pose significant hurdles. Efforts like SMART-on-FHIR apps aim to bridge this gap, though challenges persist.
❗Data Volume and Complexity
Genomic data is massive and intricate, especially with advancements like next-generation sequencing (NGS). A single test can identify thousands of genetic variants, requiring significant computational resources and bioinformatics expertise to interpret. Managing this data within existing healthcare IT systems is challenging due to its size and complexity. Normalizing such vast datasets for clinical applications, such as matching genetic profiles with treatment knowledge bases, demands extensive bioinformatics capabilities. As genomic research progresses, handling large, complex datasets will require continuous updates to healthcare infrastructure and collaboration between AI and genomics experts.
Related Read - Decoding SMART on FHIR for Healthcare Interoperability
Conclusion
Integrating FHIR with genomics is unlocking new possibilities in personalized medicine, helping healthcare providers create precise treatment plans and identify health risks earlier. This synergy offers a future where patient outcomes improve through more targeted therapies, reduced adverse reactions, and proactive care interventions, shaping the future of medicine.
HealthConnect CoPilot offers expertise in FHIR and genomics integration, ensuring interoperability with healthcare systems and delivering actionable insights for personalized treatments. By combining advanced technologies and domain knowledge, we can help healthcare providers navigate the genomics market and achieve better patient outcomes with AI-driven genomic data solutions.
- What is FHIR and how does it relate to genomics?
FHIR is a standard for exchanging healthcare information electronically. It allows seamless sharing of genomic data between healthcare systems, ensuring accurate and timely use of genetic information in clinical decision-making.
- How is AI contributing to advancements in genomics?
AI analyzes massive genomic datasets to uncover patterns, predict health risks, and personalize treatments. It helps healthcare providers use genetic insights to predict disease outcomes, develop targeted therapies, and improve patient care across the genomics market.
- What role does FHIR play in AI-driven genomics integration?
FHIR facilitates the sharing of genomic data across different platforms and healthcare systems, ensuring that AI algorithms can access and process relevant genetic information. This enhances AI's ability to deliver precise, data-driven insights for genomics.
- How does AI improve personalized treatment in genomics?
AI algorithms analyze genetic variants and pharmacogenomic (PGx) data, helping to create optimized treatment plans based on an individual’s genetic makeup. This leads to more effective therapies and fewer adverse drug reactions.
- What is the future of genomics with AI and FHIR integration?
The integration of AI and FHIR in genomics will lead to a more connected healthcare ecosystem, with real-time genomic insights driving personalized medicine. This will boost the genomics market and transform patient care through more accurate and faster data analysis.
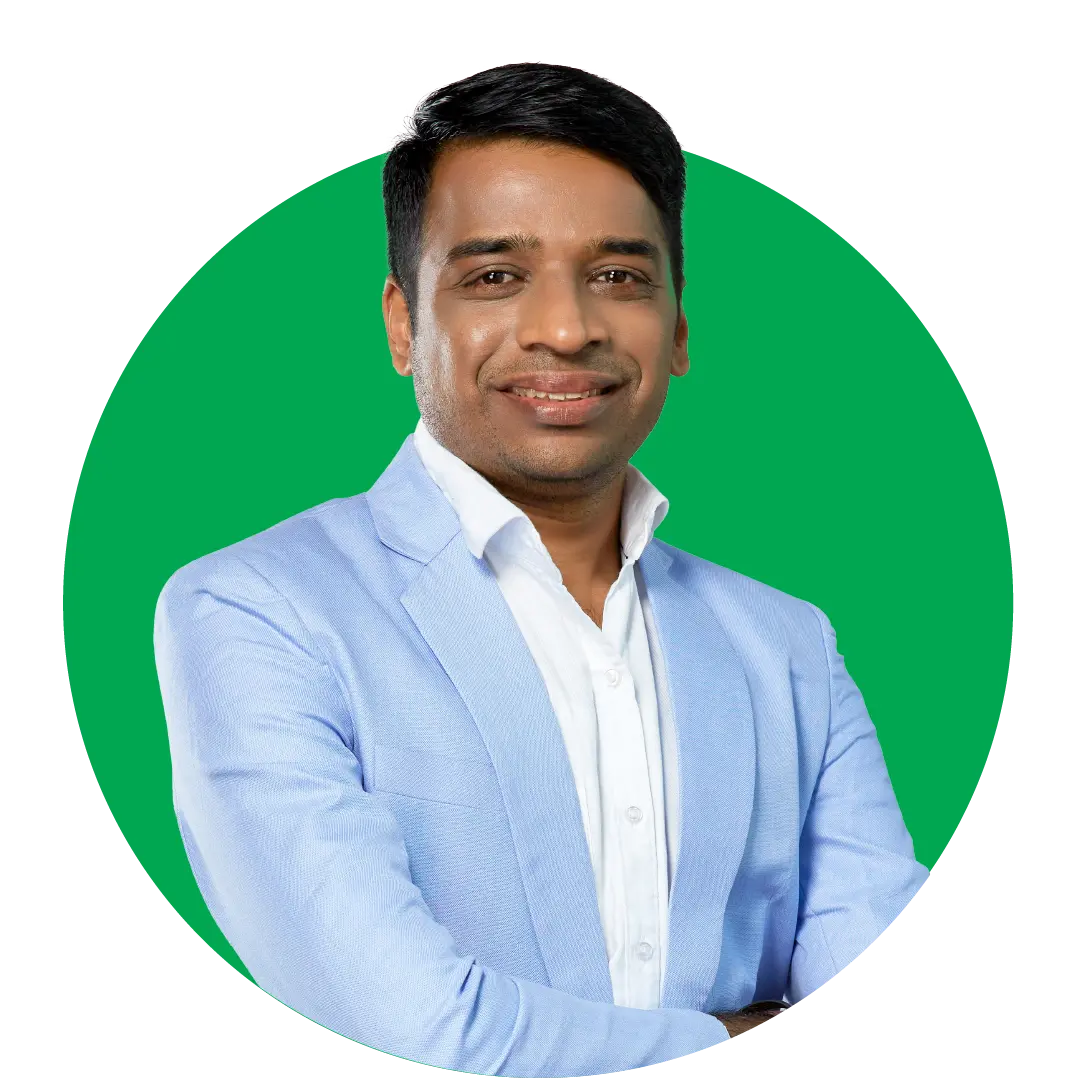
Pravin Uttarwar, CTO of Mindbowser
As the CTO of Mindbowser, a healthcare-focused software development company, I am dedicated to delivering cutting-edge digital solutions that transform patient care and operational efficiency. With over 16 years of experience and as an MIT alumnus, I specialize in healthcare interoperability, FHIR-compliant systems, and AI-powered platforms, crafting scalable products and architectures tailored to the unique needs of healthcare providers and enterprises.
I have spearheaded the development of over 100 products and platforms, guiding them from concept to full-fledged solutions. My expertise extends to scaling remote tech teams, driving EHR integrations, and building secure, cloud-native healthcare solutions. By shaping technology visions and roadmaps, I help clients achieve long-term growth and success in the rapidly evolving healthcare landscape.
HealthConnect CoPilot enabled us to access real-time patient health data through integration with Apple HealthKit, enhancing care delivery while maintaining HIPAA compliance. This led to personalized care and improved outcomes for patients.
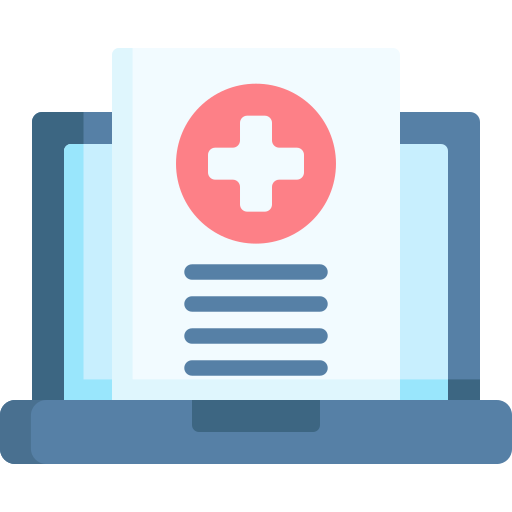
AI-enhanced Obstetrics Clinical Decision Support Platform
HealthConnect CoPilot's integration with Epic's Hyperspace has transformed our workflow. Automated post-delivery examinations and HL7 protocol use ensure accurate updates to Epic. Their expertise empowers informed decision-making in childbirth
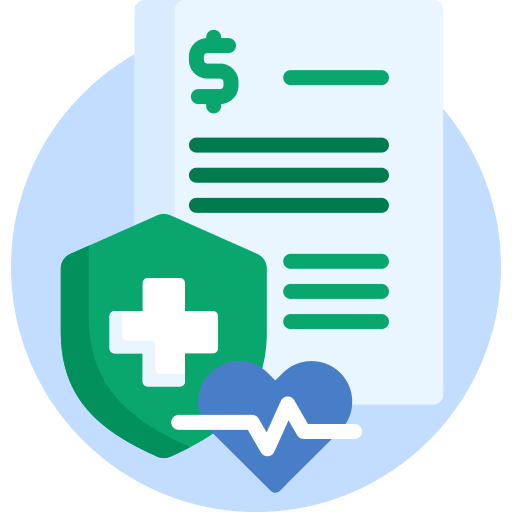
Top Provider for Customized Healthcare Solutions
HealthConnect CoPilot's helped us to integrate with leading tracking devices such as Apple Watches and Fitbit. This integration enables effortless syncing of health data, providing users with real-time insights displayed directly on our flagship products: smart mirrors and digital calendars.
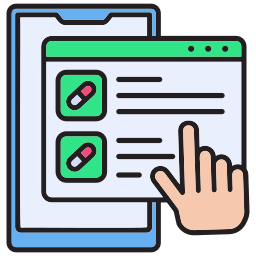
A Provider of Customizable Display Solutions
Post a comment Cancel reply
Related Posts
Checklist for EHR Integration in the Healthcare System
EHR integration in the healthcare system refers to the seamless connection of EHR systems with…
The Road to Value-based Healthcare: How Interoperability Paves the Way
Interoperability in healthcare refers to the ability of different health information systems to exchange, interpret,…
EMR vs EHR: What’s the Difference?
EMR vs EHR is a decade-old topic that makes you wonder about how different they…
How HealthConnect CoPilot Ensures Patient Data Security and Privacy
In the complex world of healthcare, managing patient data is like walking a tightrope—one wrong…
The Future of Interoperability in Healthcare
In 2018, the World Health Assembly (WHA) passed resolution WHA71.7, establishing a global strategy for…
FHIR Adoption in Healthcare
FHIR adoption is transforming how healthcare information is exchanged, offering significant benefits to healthcare providers.…